目标检测之LabelStudio
1.命令行安装
1 | pip install label-studio |
2.docker 安装
1 | # Run latest Docker version |
3.使用
首先需要注册一个账号,注册完成之后,就可以进入创建项目了。
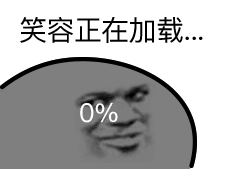
打开部署页面,然后找到,创建项目 -> 导入数据 -> 标签设置,设置完成之后保存,然后进行图片标注。这里选择矩形框的标注,然后可以增加相应的类别。
图片标注就是画框,然后选择分类,最后就提交。标注的时候,先选择下面的类别,然后在图上画一个框,修改框的时候,也是选中类别,然后调整框的大小。
保存完成之后,可以导出不同格式的数据。
【1】.Quick start 官方安装教程
【2】.Installing Label Studio 这是官方的英文,还提供了docker的数据安装
【3】.使用Label Studio标注YOLOv8数据集 这里进行了标注和数据下载,还有数据集划分。还提供了一个视频文件抽帧的代码。
【4】.标注工具——Label Studio安装与简单使用
4.自动标注
label studio还提供了一个集成自动标注的功能,实现该功能需要 label-studio-ml-backend 功能,我主要的修改是通过代码库 得到的。当然如果你直接使用这个代码仓库,那么可能更加的简单,不需要在修改 Dockerfile,不需要修改 model.py 和_wsgi.py等文件了,只需要把自定义的 best.pt 放到 data/server/models 目录下就行了。
(1)下载源码
从github上下载相关的源码,然后。
1 | # 创建虚拟环境 |
(2)创建项目
1 | label-studio-ml create ml_backend |
创建一个空的目录结构,其中 model.py 为主要的代码,其中就有 predict 和 fit 方法,通过修改这两个方法,就可以完成模型的预测和训练。
my_ml_backend/
├── Dockerfile
├── .dockerignore
├── docker-compose.yml
├── model.py
├── _wsgi.py
├── README.md
├── requirements-base.txt
├── requirements-test.txt
├── requirements.txt
└── test_api.py
接下来可以这么做,直接使用 label-studio-ml start 命令启动,或者是使用 docker 命令启动。
1 | (1).Try it out by running: |
(3)修改 requirements.txt
修改 requirements.txt,增加 ultralytics
1 | ultralytics |
(4)修改 _wsgi.py
修改 _wsgi.py 文件,根据参考资料里面的代码,将 _wsgi.py 代码修改了。
(5)将自定义的 best.pt 模型,放到 /usr/local/label-studio/label-studio-ml-backend/ml_backend/data/server/models 目录下。
(6)修改Dockerfile文件,增加了 libgl1,和 libglib2.0-0
1 | RUN apt-get update && apt-get install -y --no-install-recommends \ |
(4)修改 model.py 文件
实现预测逻辑,在 model.py 中,通过实现 predict 函数,来自定义自己的预测逻辑。
1 | def predict(self, tasks, context, **kwargs): |
实现训练逻辑,在 model.py 中,通过实现 fit 函数,来自定义自己的训练逻辑。
1 | def fit(self, event, data, **kwargs): |
修改上面的两个函数,最后形成了model.py文件
(5)启动项目
修改了代码之后,可以启动整个项目了。
1 | # 启动 |
(6)label-studio 连接自定义模型
找到 项目设置 -> Machine Learning,进行配置。
(7)自动标注
选中你要自动标注的图片, 找到任务下拉列表,点击 Retrieve Predictions,预标注这一列会由0 变为 1,检查是否需要手动修改。也可以在项目的设置菜单的 Label 按钮,直接进行标注。
【1】.自动标注好用吗?基于SAM和Label Studio搭建半自动实例分割标注平台 设置label studio使用SAM。同样也是在settings里面,点击Machine Learning,Add Model,填入模型名称,以及SAM 启动的URL,记得要将Use for interactive preannotations打开,最后点击右下角按钮Validate and Save
【2】.yolov8 与 label studio实现半自动化标注 实现自己的label studio ml backend,修改了 predict 和 fit 部分的代码,实现了自动标注。
【3】.label-studio-ml Label Studio ML后端是一个SDK,可让您包装机器学习代码并将其转换为Web服务器。然后,Web服务器可以连接到Label Studio以自动标记任务并从模型中动态检索预注释。简单来说就是从手动标注数据变成了模型预测,只需要手动微调(模型够精准,预测结果可以拿来直接训练)。
【4】.使用 MMDetection 和 Label-Studio 进行半自动化目标检测标注
【5】.YOLOv8 ML backend for the Label Studio
【6】.教程:使用 Label Studio 的 machine learning backend 进行辅助标注和训练 简单来讲,my_ml_backend.py 内主要包含一个继承自 LabelStudioMLBase 的类,其内容可以分为以下三个主要部分:1. init 方法,包含模型的加载和基本配置的初始化。2.predict 方法,用于为标注数据生成新的预测结果,其关键参数 tasks 就是 label studio 传递的原始数据。3.fit 方法,用于模型的训练,当点击页面上的 Train 按钮时,会调用此方法(具体的位置在下文会提到),其关键参数 annotations 就是 label studio 传递的已经标注了的数据。
【7】.Write your own ML backend 这是官方的自定义解释:Use the Label Studio ML backend to integrate Label Studio with machine learning models. The Label Studio ML backend is an SDK that you can use to wrap your machine learning model code and turn it into a web server
【8】.label-studio-yolov8-backend 这里是集成了 yolov8 的模型,我看了 model.py 代码,好像没有什么毛病。
【9】.Your-own-custom-YOLO-models 官方有一个例子,自带了yolo的代码,这里一步步的指导了相关的操作。cd label-studio-ml-backend/examples/yolo,
【10】.docker-compose up 服务如何后台运行
5.导入数据
如果有从其他软件进行标注过的数据,比如VOC数据,如何导入到 label studio 中呢?或者说直接导入以前已经标注好的数据。
(1) 开启本地文件夹支持
增加 LABEL_STUDIO_LOCAL_FILES_SERVING_ENABLED 和 LABEL_STUDIO_LOCAL_FILES_DOCUMENT_ROOT 配置,启用本地文件系统支持
1 | # 命令行启动 |
(2)设置项目路径
新建项目,设置路径:Settings / Cloud Storage / Add Source Storage ,Storage Type 选择 Local files。右上角 “Absolute local path” 中需要填写绝对路径,而且这个绝对路径需要是 Label Studio 启动时 LABEL_STUDIO_LOCAL_FILES_DOCUMENT_ROOT 中设置的路径的子文件夹。
配置项目目录要填绝对路径(Absolute local path)比如我的:在启动的时候,指定了 /home/data/label-studio 映射到了容器 /label-studio/data 目录,那么我 /home/projects/label-studio/fire 数据存放目录,到label-studio中配置:/label-studio/data/fire/images 目录即可。
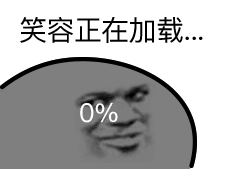
(3)测试图片访问
添加完本地存储之后,查看图片是否可以被访问。比如我的图片目录都是放到 fire/images 目录下,浏览器查看图片是否可以访问。
1 | http://192.168.1.8:10003/data/local-files/?d=fire/images/your-room1.jpg |
(4)转换voc为yolo
一般通过脚本的方式进行, objectDetectionDataset 提供了几种转换方法,可以根据需要进行修改之后使用。
1 | import xml.etree.ElementTree as ET |
(5)创建yolo目录
yolo的目录格式如下,其中 classes.txt 中,每行一个类别。通过上面的voc转换为json,然后分开各个路径。
1 | images |
(6)转换数据格式
安装 label-studio-converter,进行数据转换
1 | # 安装 |
(7)编辑 label 标签
然后在项目设置的 Labeling Interface 中,将上一步生成的 output.label_config.xml 中的内容,也填入进去。
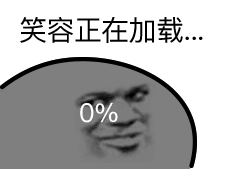
(6)导入output.json
将生成的 output.json,导入入到项目中
(7)完成
这样本地的标注项目就被导入到了 label-studio 中了,开始的时候,我的 output.json 里面没有坐标信息,导致我始终找不到标注在哪里。
【1】.pre annotation (importing pascal VOC format (xml))
【2】.标注工具Label Studio如何导入本地文件和模型预测结果 导入模型预测结果
【3】.数据标注工具 将现有标注转为json格式可以用label-studio-converter工具,安装命令为pip install label-studio-converter
【4】.label studio导入本地数据集同时邀请他人标注 在启动虚拟环境之前需要先设置环境变量允许访问本地文档,设置数据集路径,设置为数据集的上一个文件夹即可
【5】.Configuring Local File Serving for Label Studio on Docker (Windows) 这里给了解决方案
【6】.label-studio — dataset storage
【7】.label-studio-converter 这篇文章还详细提供了从yolo导入到label studio的方法,大部分可以参考。Label Studio Converter是一款开源的标注数据转换工具,可将Label Studio的标注结果转换为JSON、CSV、CoNLL 2003、COCO、Pascal VOC XML等多种机器学习格式。此外,它还支持将YOLO格式的预标注数据导入Label Studio。通过命令行和Python API接口,Label Studio Converter简化了数据格式转换流程,有效连接了数据标注和模型训练环节。操作步骤:1.设置环境并运行Label Studio;2.设置本地存储;3.验证图像访问;4.转换YOLO标注;5.导入转换后的标注;6.验证标注;
【8】.【最全教程】VOC格式转YOLO格式数据
【9】.Import pre-annotated data into Label Studio 这里是官方的说明,但是好像不是很明白。
问题
1.PermissionError: [Errno 13] Permission denied: ‘/label-studio/data/media’
【解决方案】
1 | chmod 777 -R label-studio |
2.ModuleNotFoundError: No module named ‘ultralytics’
在使用docker启动 ml backend 的时候,总是报错。
【尝试方案】
(1)我在 ml_backend 执行了 pip 安装了 ultralytics,无效。
(2)修改了 requirements.txt、requirements-test.txt、requirements-base.txt,增加了 ultralytics。requirements_base.txt and requirements_test.txt are basic dependencies (you don’t need to modify this)
(3)修改了 Dockerfile ,增加了清华源,也是无效。
1 | RUN pip config set global.index-url https://pypi.tuna.tsinghua.edu.cn/simple |
【解决方案】
后来的解决方案,我觉得可能是用的 –build 参数。
1 | docker compose up --build |
【1】.Dockerfile换apt源和pip源
【2】.YOLO ML backend for Label Studio Step by step guide: Using your own custom YOLOv8 model
3.error: RPC failed; curl 16 Error in the HTTP2 framing layer
执行 docker 启动 ml backend之后,出现了:executor failed running [/bin/sh -c pip install -r requirements-base.txt]: exit code: 1。意思就是:Running command git clone –filter=blob:none –quiet https://github.com/HumanSignal/label-studio-ml-backend.git /tmp/pip-install-y189u4fc/label-studio-ml_6fc0e90d1fec4709a7be0f377235b3cd
31.72 error: RPC failed; curl 16 Error in the HTTP2 framing layer
这里其实就是从 label-studio-ml-backend.git 进行安装
【尝试方案】
因为label-studio-ml-backend项目就是我从github上下载下来的,于是我就修改了requirements-base.txt,从 https 上下载,改为了直接引用本地仓库,但是没有成功。
1 | # label-studio-ml @ git+https://github.com/HumanSignal/label-studio-ml-backend.git,改为: |
【解决方案】
我也没解决,就是删除了创建的 ml-backend,然后重新安装的。
我感觉特别的奇怪,有时候可以,有时候又不可以,多试几次就可以了。
【1】.pip git+install 报错解决 对于已经安装git 的同学,可以 git clone 网络地址,cd 到下载的文件,再 pip setup.py install
【2】.pip install from git repo branch pip install -U git+https://github.com/danreeves/wagtailgmaps@3.0.0
【3】.https://blog.axiaoxin.com/post/python-requirements-file/ requirements.txt 文件格式详细说明
【4】.requirements.txt 文件声明依赖 除了 git 协议,pip 还支持以下传输协议:git git+http git+https git+ssh git+git git+file
【5】.The git+file requirement format doesn’t seem to work the way documentation says it does
4.libGL.so.1: cannot open shared object file: No such file or directory
这个问题其实是我注释了上面的requirements-base.txt, 然后执行导致的。
1 | label-studio-ml @ git+file:///usr/local/label-studio/label-studio-ml-backend, |
【尝试方案】
(1)我也没解决,就是删除了创建的 ml-backend,然后重新安装的。
(2)我也尝试了修改了 requirements.txt,增加了 opencv-python-headless,结果无效
(3)修改了 Dockerfile 文件,增加了如下的代码,结果 Unable to locate package libgl1-mesa-glx
1 | RUN apt install -y libgl1-mesa-glx |
(4)修改 Dockerfile 文件,增加了 下面的代码
1 | RUN apt-get update && apt-get install -y python3-opencv |
【解决方案】
修改了 Dockerfile 文件,增加了 libgl1,libglib2.0-0。
1 | RUN apt-get update && apt-get install -y --no-install-recommends \ |